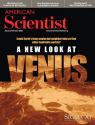
This Article From Issue
January-February 2021
Volume 109, Number 1
Page 54
DATA FEMINISM. Catherine D’Ignazio and Lauren F. Klein. 324 pp. The MIT Press, 2020. $29.95. (The book can be read free of charge online.)
As scientists, we may have an inclination to view data as scientific facts that speak for themselves. But data are the product of many small but cumulative choices we make about what to document and quantify. Those choices are important, because data have the potential to be enormously influential. In Data Feminism, Catherine D’Ignazio and Lauren F. Klein invite us to think deeply about the underlying forces that can subtly shape the presentation and analysis of data, and about the conclusions the data are used to support. They propose that we use feminist thought to guide our approach. The book includes many examples of data science relating to sex and gender, but don’t let the title fool you—the authors’ recommendations apply to all data and all kinds of science.
Data feminism is ultimately about power—about who has it and who doesn’t, and how power imbalances can be shifted. Data have power that is often wielded uncritically, without considering whose agenda is being served. Data feminism attempts to correct that, in large part by expanding awareness of implicit biases in the collection and presentation of data. According to the authors, the feminism that informs data science needs to be intersectional—that is, it should consider how additional dimensions of identity, such as race and class, intersect with feminism and with each other. D’Ignazio and Klein are using the term feminism, they say, as a “shorthand” for “projects that name and challenge . . . forms of oppression [and] seek to create more just, equitable, and livable futures.” Their aim is to examine inequities and use data to make change.
Data Feminism is also about and for members of communities that have been minoritized (devalued and disadvantaged by dominant groups); the authors believe strongly that minoritized people and communities need to be involved in decisions about what data should be collected and how those data should be used and presented. D’Ignazio and Klein intentionally use an expansive definition of data, in part to push back against historical gatekeeping in science that has focused on asking “What are ‘real’ data?” and “Who is doing ‘real’ science?”
The book is organized into seven chapters, each of which in turn takes up one of seven principles: (1) “examine power” (consider the current configuration of society, in which dominant groups experience unearned advantages and other groups are disadvantaged); (2) “challenge power” (analyze and expose oppression, envision equity); (3) “elevate emotion and embodiment” (remember that visualization is rhetorical and that unemotional visualizations are not neutral); (4) “rethink binaries and hierarchies” (question how things are counted and classified); (5) “embrace pluralism” (include multiple perspectives and the voices of the minoritized at all stages of a data project); (6) “consider context” (remember that numbers derive from a data setting that has been influenced by power differentials); and (7) “make labor visible” (acknowledge to the greatest extent possible the vast network of people who have contributed to a data project). Each chapter demonstrates the importance of its principle and provides examples of organizations or scholars applying the principle in their approach to working with data. The authors’ goal is to explain, and advocate for, a process-based approach.
Power can take the form of refusing (or neglecting) to include a particular group when data are being collected. One way to respond is with counterdata—data that individuals and grassroots organizations collect and analyze themselves in order to combat existing power imbalances. Some of the most interesting examples in the book have to do with counterdata. For example, more than 50 years ago in Detroit, Black residents of neighborhoods alongside a popular commuter route through the city became concerned at the number of their children who were being killed when hit by the cars of commuters. They couldn’t find any data to substantiate what they were seeing, because detailed records of these deaths were not being kept, so they decided to collaborate with white male geographers at nearby universities to produce a heartbreaking map of this vehicular violence, titled “Where Commuters Run Over Black Children on the Pointes-Downtown Track” (1971); the map used black dots on a street grid to show where children had been killed. More recently, the Westside Atlanta Land Trust used participatory data collection to build its own set of data about abandoned properties and property disinvestment and used it to lobby municipal policy makers for affordable housing.
Yet the authors make the point that collecting data on minoritized groups isn’t necessarily a good thing in and of itself: Scientists must take care not to subject such groups to excessive surveillance and data collection. The book discusses this in connection with facial recognition software. When such software was shown to have difficulty recognizing darker-skinned female faces, it was found that this was because the data set on which the software algorithms were trained consisted largely of the faces of white men. Projects aimed at remedying this problem have been compiling databases with greater diversity of faces. Unfortunately, software with an improved ability to detect faces of color can be used by governments and police forces to oppress people of color. This is of particular concern in countries that have poor human rights records, such as Zimbabwe and China, which recently struck a deal to share facial recognition information and technology.
Overall, D’Ignazio and Klein argue for what’s known in anthropology as studying up, the too-rare practice of people with relatively little institutional power studying those higher up in the power structure. For example, instead of studying a community affected by pollution, researchers could study the polluters. In the context of data feminism, studying up means intentionally collecting and using data to interrogate and challenge those with power, while refusing to participate in data collection that harms minoritized people and communities. An example of data collection that harms such groups is a widely used algorithm that assesses the risk that someone who has been arrested will commit a future crime; in 2016, an investigation by ProPublica determined that Black defendants were more likely than white defendants to be mislabeled as high risk by the algorithm and to be denied bail as a result.
Questions about which groups should be represented in data collection are important, but it is even more important to ask who benefits from the data. It may shock some readers to learn that the authors are not necessarily fans of initiatives to promote “data for good” (projects undertaken in the public interest) and initiatives to promote “data ethics.” (Data ethics initiatives are promising, they say, but often simply serve as a Band-Aid.) According to D’Ignazio and Klein, both of these frameworks fall short when it comes to shifting power to minoritized people and groups; they therefore urge data scientists to focus on equity rather than fairness, and on justice rather than ethics. They aren’t trying to shame anyone; they are simply suggesting ways of distinguishing between projects that aim “for good” and those that aim for liberation. In projects using data for liberation, members of minoritized groups lead the project from the start; they also own the data collected and participate in its analysis, with data scientists acting as facilitators and guides.
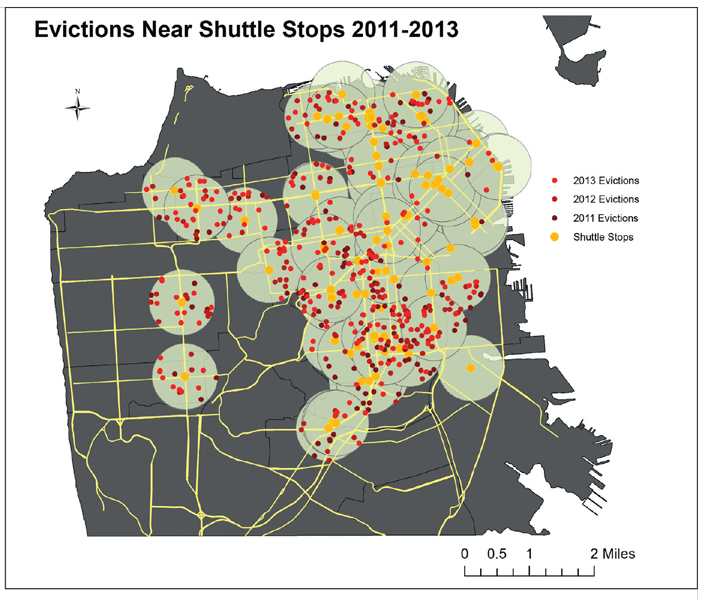
From Data Feminism.
A series of such projects discussed in the book have been carried out over the past several years in the San Francisco Bay area (see figure above) by the Anti- Eviction Mapping Project, which consists of “housing justice activists, researchers, data nerds, artists, and oral historians”; some of these projects have involved collaboration with the Eviction Defense Collaborative, which provides court representation for people who have been evicted. The two groups collect and share systematic data on eviction trends; the goal is not merely to raise awareness but to bring about change.
Data Feminism is focused on solutions. For instance, to help ensure that data are not reused inappropriately, the authors recommend adding context in the form of “data user guides,” a concept developed by Western Pennsylvania Regional Data Center. The guides explain the details and purpose of the data collection and may include caveats about the limitations and a discussion of the ethical dimensions of the data. The book also provides many examples of how to “show your work” in ways that give credit to the many people and kinds of knowledge needed to carry out data projects. For example, the authors propose that GitHub repositories of open source code be designed in a way that allows viewers to see all types of contributions to the production of code.
The emphasis in the text on process is matched by the process the authors undertook to create the book: They put a draft of the manuscript (akin to a preprint) online and invited community peer review. The draft and public comments on it are still available online. A side-by-side comparison with the finished book reveals that several sections contain additions that were suggested by the community reviewers; one such addition recommends moving beyond a gender binary when discussing invisible labor. The final text of the book includes appendixes that contain the authors’ guiding values and the metrics they used to measure their success in addressing structural problems such as racism in both the public draft and the printed book. In this regard, the book is an excellent example of the process that it encourages data scientists to use.
Data Feminism covers challenging topics such as structural racism and sexual violence, but it is narrative-driven: The authors make their points by telling detailed stories about how various data projects have been carried out. Free of jargon and easy to read, the book can be enjoyed by scientists in many different fields.
The text of each chapter is complemented by a number of instructive data visualizations—charts, graphs, photos, maps, and other figures. A number of these are nontraditional, and some are visually quite compelling. For instance, a figure from the book, titled “Bruises—the Data We Don’t See,” depicts the emotional journey of a mother (Kaki King) whose daughter (Cooper) has been diagnosed with idiopathic thrombocytopenic purpura, a disease that presents as bruises and petechiae (minute reddish spots on the skin containing blood). The figure records changes to the daughter’s skin, accompanied by a record of the mother’s feelings as a caregiver. Each day is represented by a white aspen-shaped leaf colored with purple splotches to show intensity of bruising and pink dots representing petechiae (see detail from the figure below). Curved purple and orange lines alongside the leaf represent, respectively, the mother’s fears and hopes, as do her comments, which range from “devastated” to “today I just wanted her to have fun.” The figure uses an expansive definition of data and embodies the principle that emotion should be elevated.
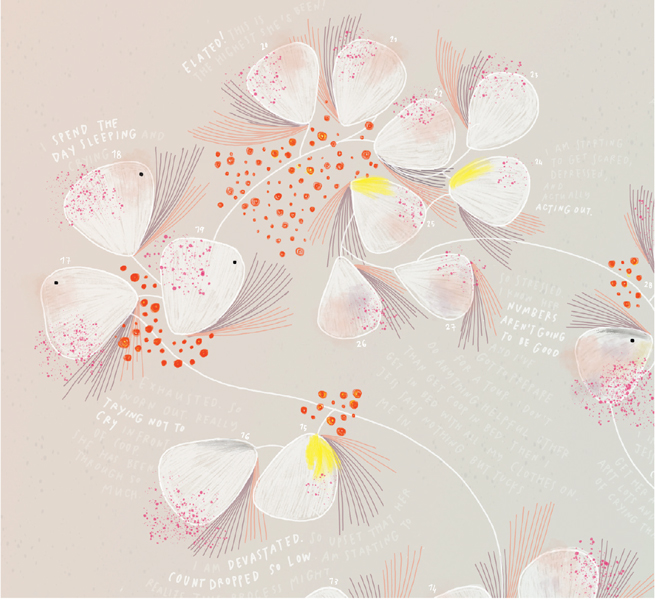
From Data Feminism.
Unfortunately, this striking visualization is the only one in the entire book that refers explicitly to disability, and it appears to violate one of the credos of disability advocacy—“Nothing about us without us.” A shortcoming of the book is that the authors’ only metric for holding themselves accountable for addressing ableism (discrimination or prejudice against people with disabilities) is the inclusion of nonvisual methods of data presentation, and the text does not indicate whether blind individuals were involved in the creation of the examples of nonvisual elements provided.
Another topic that I wish the book had given more attention to is Indigenous data sovereignty—methods of using data in ways that respect the right to self-determination of Indigenous communities. Data about members of those communities should not be collected, kept, or used without their consent and leadership.
Anyone who works with data—and all scientists do, of course—will benefit from reading this book. But the readers who may gain the most from it are those who are trying to use data in the public interest. Data Feminism does such a good job of integrating theories and projects across several fields that it will likely become a touchstone for teaching data science that goes beyond data ethics.
American Scientist Comments and Discussion
To discuss our articles or comment on them, please share them and tag American Scientist on social media platforms. Here are links to our profiles on Twitter, Facebook, and LinkedIn.
If we re-share your post, we will moderate comments/discussion following our comments policy.