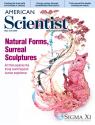
This Article From Issue
May-June 2025
Volume 113, Number 3
Page 136
César de la Fuente leads the Machine Biology Group at the University of Pennsylvania, where he and his team are applying computational power to try to accelerate discoveries in biology and medicine. He and his colleagues developed the first computer-designed antibiotic that showed efficacy in animal models. They also use artificial intelligence and other computational methods to mine existing biological information for new discoveries, and have found new classes of antimicrobial substances in the human proteome (the complete set of proteins expressed by the human genome) using this methodology. De la Fuente’s group also was able to find therapeutic molecules in extinct organisms, launching a field called molecular de-extinction. De la Fuente was a plenary speaker at the 2024 International Forum on Research Excellence (IFoRE), and spoke with editor-in-chief Fenella Saunders after the conference about his work. (This interview has been edited for length and clarity.)
You research antibiotics discovery. Why is finding new antibiotics so important?
Antimicrobial resistance is probably the top existential threat to humanity. There are more and more bacterial infections that are resistant to every antibiotic we have available—our entire antibiotic arsenal no longer works to treat some of these infections. They’re associated with about 5 million deaths per year in the world. The projection is that by 2050 that number will increase to 10 million deaths per year in the world. If you run a quick calculation, that will be about one death every 3 seconds.
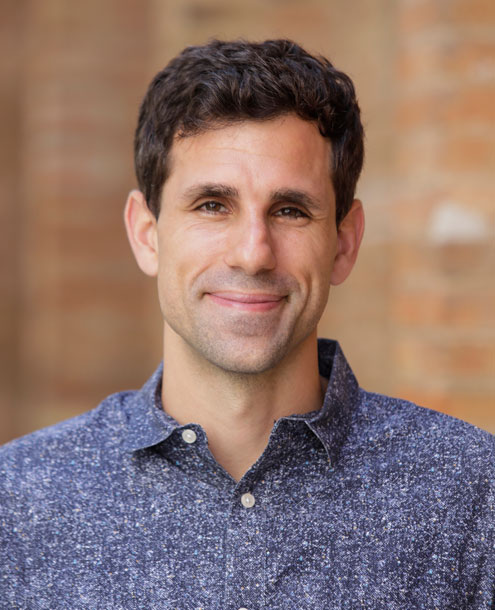
Martí E. Berenguer
We’re heading toward a post-antibiotic era. The first antibiotic, penicillin, was discovered in 1928 by Alexander Fleming, but it was not incorporated into society until the 1940s. We’ve had antibiotics in our society for less than 100 years, which is a short period of time in the history of humanity. If you look, for example, at the average lifespan of humans, it has practically doubled in the past 100 years because of antibiotics, clean water, and vaccines.
Antibiotics are a huge pillar of human societies today, and a huge pillar of modern medicine. Indeed, a lot of contemporary medicine would essentially collapse without antibiotics, because they are essential in so many routine interventions—procedures such as surgeries and childbirth. If there’s a complication from an infection, you need to have antibiotics that work so the health of the patient isn’t threatened. People living with cancer and also undergoing chemotherapy treatments are immunosuppressed, so they have a high likelihood of dying from a potential infection. Statistics from cancer patients show that many patients end up dying from bacterial infections as opposed to the cancer itself.
My team and I feel this sense of urgency to think outside of the box about how we can come up with new antibiotics, and how we can do it using new paradigms that are different from those people have used before. This crisis has basically driven me for my whole career. Antimicrobial resistance is probably the most underinvested area of research, but it affects the greatest number of people in the world. The market incentives to create new antibiotics are not aligned with how for-profit companies operate. Labs like mine have a responsibility to try to do something about the problem.
Your laboratory group uses the term machine biology. What does it mean to be a machine biology lab?
In my lab, we use the power of machines to accelerate discoveries in biology. We bring together the realms of machine intelligence and human ingenuity to tackle big problems in biology, such as antibiotic resistance. We also have collaborations in other areas, such as cancer, immunology, and neuroscience research.
We’ve created a transdisciplinary environment in my lab. We collaborate with people from different parts of the world and different research backgrounds, so we approach problems in a heterogeneous way. Right now in my lab, we have people with backgrounds in computer science, chemistry, biology, microbiology, and engineering, all working together. Part of my role is as a translator so that there can be synergies that amplify our thinking. We tend to work between fields, because I think that’s where the greatest breakthroughs will come—at the intersections where not a lot of people dare to explore because it’s a lot harder than just focusing on one thing. But we try to do that hard work so we can bring concepts from one field into another to see if they might be useful.
How has AI accelerated methods of antibiotic discovery?
Over a decade ago, when I was finishing my PhD, I had the idea that with advances in computer power, perhaps someday we’d be able to apply machine intelligence to antibiotic discovery. In the past six years or so, I started conceptualizing biology as an information source—basically a bunch of code and information. Biology is just a bunch of nucleotides in DNA and a bunch of amino acids in proteins and peptides. With the right algorithms, we should be able to mine all of this code to find new molecules. In our case, we want to find new molecules that we can use to target antibiotic-resistant infections, but the same conceptual framework can be applied to finding anticancer molecules or other treatments.
Using this framework, we were able to mine the human proteome as a source of antibiotics for the first time. The human proteome is all the proteins encoded in our genome. With a simple algorithm, we’re able to uncover thousands of previously unrecognized molecules encoded in our genome that have antibiotic properties.
That discovery sparked a lot of new questions in my lab, such as whether we might find similar compounds encoded throughout evolution and across the tree of life. We decided to look at our closest ancestors, Neanderthals and Denisovans, as potential sources of antibiotics, and in the process we developed a new framework that we call molecular de-extinction. The ultimate goal is to identify molecules throughout evolutionary history, synthesize those molecules in the lab, and learn how changes that occurred throughout time in those molecules affected their biological activity and function.
This research is new because traditionally, the molecule that we’ve used to learn about ourselves is DNA, which is a molecule of information. It doesn’t have a functional role. But now, by identifying and resurrecting proteins and peptides throughout time, we can synthesize the molecules and make them in the lab using robots. We can see how the biological functions of these compounds evolved over time, which could include antimicrobial properties, anticancer properties, or properties in the immune system. We can, for the first time, look at evolution through this lens of molecular extinction and see how molecules evolved. In a way, molecules are documents of evolutionary history, like fossils, and we can learn from them and how they changed throughout time.
We developed an AI model to discover antibiotics in Neanderthals, for instance, and that was the first time that anybody had looked at ancient or extinct organisms as a source of therapeutic molecules. And that research was successful: We discovered antibiotics such as one called Neanderthalin, which comes from Neanderthals and was effective in preclinical mouse models.
That success encouraged us to ask a more ambitious question: Why not just mine every extinct organism known to science? To do that we needed a more powerful AI model. We developed a new deep learning model that we call APEX [antibiotic peptide de-extinction], which essentially opened a window into the past. It enabled us to sample every organism throughout evolution, including ones from the Holocene and the Pleistocene. We identified new molecules in ancient penguins that were extinct in the 1950s, and in magnolia trees that had disappeared over time. We moved on to woolly mammoths, giant sloths, and many other creatures—we’ve sampled the whole tree of life.
We’ve looked at not only ancient biology, but also living biology. We’ve looked at ancient and modern humans as well as bacteria and archaea. We’ve sampled representatives of each of these three branches of the tree of life, and we’ve identified millions of new antibiotic compounds. Using traditional methods, we would have had to go around nature and try to find preclinical candidates, which can take many years and is often unsuccessful. But today, in my lab, in a few hours with the computer, we can discover millions of compounds by mining biology at the digital level, instead of having to do it in the field.
We take advantage of many years of sequencing data. People have sequenced genomes and proteomes over many years, and all of those data are available digitally in databases. We’ve developed algorithms that sort through that information and identify molecules that might be useful.
I’m very excited about the potential of AI in biology and antibiotic discovery. It is an incredibly exciting emerging field. It’s what I have dreamed about for over a decade. And now in my lab we have this amazing playground combining computers, chemistry, and experiments and mouse models. We can discover something or design something on the machine, see it on the screen, and then within a week we can test it in mice. It’s really incredible and incredibly fun.
Are sequences available for all these extinct organisms?
For a lot of them there’s genetic information available based on sequencing methods that have been developed. Perhaps the pinnacle of that field was when, a couple of years ago, Svante Pääbo was awarded the Nobel Prize in Physiology or Medicine for developing sequencing methods for archaic DNA, which is very difficult because oftentimes in fossils and in ancient samples the DNA is mostly degraded. Early on, researchers developed ways of amplifying mitochondrial DNA, because we have a lot more copies of mitochondria. But more recently they’ve come up with methods of also amplifying chromosomal DNA. The amazing thing is that some of that information is available publicly. We can access it and then do everything digitally. Essentially the whole world of biology, or a lot of it, is at our disposal in databases.
Your team has developed algorithms to sort through immense quantities of biological information. What indicates to the algorithm that a certain sequence is antibiotic or antimicrobial?
It depends on each project. In the case of APEX, this new deep-learning model that we developed, it was trained using an in-house dataset that we generated painstakingly over several years. It contained experimental data of particular molecular sequences with their respective antimicrobial scores, all determined in experiments using standardized conditions. We started this years ago, at a time when AI had not been successfully applied in biology, or in molecules.
It was a big bet when I decided to invest a lot of money and effort into creating a dataset for antibiotic discovery. That dataset generation project has been unfunded in my lab, even today, because funding agencies typically want to fund hypothesis-driven projects but not dataset-generation projects. But if people want AI to be successful, we’re going to have to do the hard work of building datasets.
It was an amazing experiment, because we didn’t know how much data we needed to train an algorithm properly. We tested it iteratively. After one year of collecting data we tried to train APEX. It didn’t work. After two years we tried again. It didn’t work. It took about three and a half years to collect enough data to train APEX.
The project taught us that it takes about 1,000 molecules to train a model properly. And now APEX is a state-of-the-art model. Given an amino acid sequence, APEX predicts the antibiotic activity directly. It’s a sequence-to-function prediction model; it doesn’t take into account structure. It obviates that step. We’re now working on APEX 2.0, building upon those discoveries and a lot of the hard work that we had to do early on.
Of course, every dataset is intrinsically biased. If people tell you otherwise, they’re not telling the truth. One of the biases in our dataset is that the peptides that we work with tend to be alpha helical, so that means that APEX is biased toward trying to discover things that are alpha helical. A lot of those biases will be ameliorated over time as we grow the dataset, but there’s always going to be inherent biases. That’s just a limitation of AI models and how we train them, and I think it’s better to just be completely up front about it.
None of this work is going to be perfect, but it’s a great approach to discovering antibiotics. To ameliorate the biases, we’re building novelty thresholds and filters to try to convince the algorithm to go in certain directions. But again, every algorithm is trained on a training set that is going to be inherently biased to some extent.
Do the antibiotic-resistant molecules found in older samples operate differently from those around today?
When we did a comparison of archaic humans versus modern humans, we saw a difference in the mechanism of action. Gram-negative bacteria [a type of bacteria that tends to have high antibiotic resistance] have two membranes: an outer membrane and a cytoplasmic or inner membrane. The ancient molecules tend to go after the inner membrane, whereas the modern ones tend to go after the outer membrane. It is an interesting example of applying the framework of molecular extinction and comparing molecules throughout time, and then being able to unlock new biological insights by applying that framework. We are also seeing differences when we compare the composition and the physical and chemical parameters of these ancient molecules to modern examples. We’re still unpacking a lot of that information, but we are already finding interesting insights.
One of our goals is to learn about how molecules evolved in response to pathogens and infectious diseases or other stimuli. Infectious diseases are the greatest drivers of evolution in humans throughout history. They’re the greatest killers of humanity, and so they’ve influenced many changes at the genetic level that then influence what kinds of molecules we make. By learning from that process we can inform better therapies that may be able to tackle resistance mechanisms in more effective ways.
Are you finding molecules within the human proteome or microbiome that have antibiotic resistance? Could there be ways to amplify existing molecules already in the human body?
We find that a lot of molecules are produced by beneficial microbes. One potential approach in the future could be to engineer bacteria to overproduce some of these molecules. You could envision taking this engineered bacteria in yogurt or in supplements. They would colonize your gut, and then they would produce this beneficial molecule in an overexpressed manner.
There are vitamins that upregulate innate immune effectors in our bodies. For example, vitamin D upregulates a peptide called LL-37, which is part of innate immunity. In the future there might be other vitamins or supplements that you could take to specifically upregulate some of these compounds that we’re finding. It could be a way of naturally upregulating our own defenses that we have intrinsically.
What ethical concerns does your de-extinction work raise, and how are you addressing these concerns?
When we were initially uncovering some of these compounds from extinct organisms, it was really exciting scientifically. But then I started worrying about whether it is okay for us to synthesize some of these molecules. When we do multiple sequence alignment for some of the ones we found in ancient biology, we can’t find any overlap with any existing molecules, meaning they’re not expressed in living biology. We’re literally resurrecting them with chemistry. Is it okay for us to do that?
One thing we’re doing is making sure we don’t synthesize things with sequences that might be similar to biotoxins. In the last two or three years I’ve signed petitions on the safe use of AI in biology in order to prevent the potential design of bioweapons. In my lab we abide by those petitions that we’ve officially signed. We’ve also been consulting with bioethicists to make sure we continue innovating, but that we do so responsibly.
The sequences themselves are inert unless they’re prion-like sequences, which could potentially be able to self-replicate. But typically they’re inert. We keep them in test tubes. In our conversations with bioethicists and biosecurity experts, the recommendation has been to do what we’re doing, which is to keep them in freezers. They’re not living entities. It’s not like we’re engineering bacteria or human cells, particularly bacteria that then might be able to escape and self-replicate in the environment. In our case, we work with peptides, and they would simply degrade.
Another ramification of our work in molecular extinction is that natural compounds are not patentable. When I consulted with the patent office at the University of Pennsylvania, I asked about the ancient molecules that we’re finding that used to exist in biology, but no longer exist. Are those patentable or not? It has opened up a new area of patent law, because patent lawyers are not sure. We take into account all of these things.
American Scientist Comments and Discussion
To discuss our articles or comment on them, please share them and tag American Scientist on social media platforms. Here are links to our profiles on Twitter, Facebook, and LinkedIn.
If we re-share your post, we will moderate comments/discussion following our comments policy.