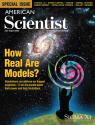
This Article From Issue
July-August 2023
Volume 111, Number 4
Page 194
Modeling is a technique that cuts across scientific fields and uses many different technologies. A mathematical model can be used to study climate, or galaxy formation, or cancer. An animal model can reveal new insights about a disease. Models sometimes are employed to interpret processes involving subatomic particles, such as neutrino detection or nuclear fusion. Models can be used to examine the past, such as the process of human evolution, or they can be used to try to predict the future, such as in global demographics.
Although models are ubiquitous in science, they are not a magic box, and they are only as good as we make them. Properly conceptualizing them is an art as well as a science, and their limits of applicability have to be carefully considered. In addition, scientists have to be vigilant in creating models that don’t inadvertently introduce bias, especially when artificial intelligence is employed in attempts to cope with the mountains of data that a model can use and produce.
In this special single-topic issue, we take a step back and give the big picture of what models can and can’t do well. In Approximating Reality, Kevin Heng of Ludwig Maximilian University in Germany kicks off the discussion with his reflections on what it means to understand a concept in the natural world, and how that definition of understanding can affect how a model of a phenomenon is built. Producing the most complex model, Heng notes, may not always be the goal. As he says: “We simulate in order to mimic as much of an observed phenomenon as possible. But we achieve understanding by using idealized models to capture the essence of the phenomenon.”
Heng says that AI could be a boon to modeling, because automating the process of designing, writing, and interpreting models could help scientists figure out direct causation of effects more efficiently, allowing them to spend more time formulating deep, creative questions for the models to answer. But in Bias Optimizers, Damien Patrick Williams of the University of North Carolina at Charlotte focuses more on the creation of AI and the inherent biases that it can amplify, making it difficult to root out even unintentional sources of problematic results.
There are many cases in which data selection can affect model performance. In Building Better Growth Curves, William E. Bennett, Jr of Indiana University School of Medicine discusses the history of infant growth projections and why they can often lead to false positives when diagnosing children with what’s called failure to thrive. But he also explains that efforts to personalize growth curves can raise serious concerns about data privacy and implicit bias.
Anne Goujon of the International Institute for Applied Systems Analysis in Austria discusses how historical demographic data can help countries plan for the future, in Predicting an Aging and Changing World. Goujon describes models of Niger’s future population that are based on whether or not funding is increased now for education, and explains how these different models can help policymakers decide where to use their limited resources to achieve the most prosperous outcomes for their countries.
Readers can find lots more in this issue, and additional online content can be found on our blog page. Join us on social media to share your own experiences with modeling.
—Fenella Saunders (@FenellaSaunders)
American Scientist Comments and Discussion
To discuss our articles or comment on them, please share them and tag American Scientist on social media platforms. Here are links to our profiles on Twitter, Facebook, and LinkedIn.
If we re-share your post, we will moderate comments/discussion following our comments policy.