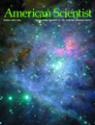
This Article From Issue
March-April 2002
Volume 90, Number 2
DOI: 10.1511/2002.10.0
Although the scientific rationale for the International Space Station has been hotly debated, few would disagree that its construction amounts to an engineering marvel. Over the past three years, 16 countries have contributed material and personnel to assemble in orbit a structure weighing, well, weighing nothing but with a mass that will eventually exceed 400,000 kilograms. So it's no great wonder that NASA wanted this momentous engineering endeavor to be well documented. One result will be a wide-format 3-D movie on the subject, due to be released this spring. It is just one example of the modern marriage between big science and the big screen.
Anyone who has seen IMAX movies in 3-D will testify that the experience is nothing like seeing a 1950s horror film using paper glasses with color filters. The current technology relies on headgear with special windows that contain electronic shutters, one for each eye. The rapid-fire opening and closing of these shutters is synchronized to the frames of film being projected in such a way that right and left eyes see camera perspectives obtained from slightly different angles, thus achieving the desired stereoscopic effect.
The Space Station movie, which is being sponsored by Lockheed Martin, was made under a so-called Space Act agreement between NASA and IMAX Corporation. Similar compacts governed the production of four other IMAX space documentaries, and indeed these agreements have been used to formalize collaborations between NASA and the producers of such commercial films as Warner Brothers' Space Cowboys and Disney's Mission to Mars.
The agreement governing the making of the Space Station movie was signed in May of 1997. According to Paula Cleggett, NASA's deputy associate administrator for public affairs, "No money is changing hands." So the arrangement is fundamentally different from the one Ron Howard had when he rented NASA's KC-135 jet—the "Vomit Comet"—to film scenes of weightlessness for the 1995 blockbuster Apollo 13. In this case, NASA charged IMAX nothing to install in the cargo bay of the Space Shuttle a remotely controlled wide-format camera during three missions conducted between December of 1998 and July of 2001. The agency also offered to ferry a bulky IMAX camera and special lighting to film the interior of the Space Station and trained its astronauts to act as cinematographers, directors, sound mixers and lighting technicians. In return, NASA retained copyright to all the footage shot (which it promises eventually to release publicly) and gained an opportunity to reach out to a vast audience of moviegoers in 30 countries.
Science buffs who thrill to this virtual ride up into space will probably also enjoy another IMAX documentary coming this summer—one that takes them down to the seafloor. For this feature, entitled Volcanoes of the Abyss, IMAX cameras followed scientists to hydrothermal vents at the bottom of the Atlantic and Pacific Oceans—or rather, it was the scientists who followed the IMAX cameras, the expeditions having been formulated expressly to obtain the wide-format film footage. The making of the documentary was funded in part by the National Science Foundation's Division of Elementary, Secondary and Informal Education.
The film (frames of which can been seen in the article "Life After Death in the Deep Sea," published in the September–October 2001 issue of American Scientist) is said to be spectacular. Indeed, Rita Colwell, director of NSF, thought it so impressive that she showed some early clips when she testified before the Senate about NSF's 2001 budget request. But unlike other IMAX science documentaries, Volcanoes of the Abyss promises to bring scientists advantages beyond favorable public relations. It may well benefit investigators directly by allowing them extraordinarily detailed views of the seafloor around hydrothermal vents, ones that had been virtually impossible to obtain previously—even for those privileged enough to have visited these sites in a deep-sea submersible vehicle such as Alvin.
Margaret Leinen, an oceanographer at the University of Rhode Island, explained the situation neatly at a symposium sponsored by the Office of Naval Research. "In Alvin," she said,"you're sort of cramped into a pretzel, looking out the bad view—because, of course, the pilot gets the good view—and you spend your entire time freezing, trying to see what is happening or guess what might be happening out the pilot's window." In noting the reaction of her fellow scientists to a special screening of the IMAX film, she said, "Everyone in the room, including the Alvin pilots, said it's much better than being in the [vehicle]."
Richard Lutz, director of the Center for Deep-Sea Ecology and Biotechnology at Rutgers University, led the effort to obtain this IMAX coverage of deep-sea hydrothermal vents. He explains that the wide-format film and high-definition video he and his colleagues obtained was of such excellent quality because Alvin was carrying some 4,000 watts of lighting: "the equivalent of sending a whole stage set down." This past December, he arranged another special showing at a meeting of American Geophysical Union. Because the screening was scheduled for 9 a.m. on the first day of the week-long conference, Lutz expected a small turnout. Instead, he reports, "The fire marshal had to turn them away at the door."
Lutz says the preparations that made the IMAX filming possible have already yielded some scientific payoffs for him as a marine biologist. And he has been approached by specialists in other disciplines hopeful that they, too, might mine the new images for their own research. Although offering this material to the scientific community was always a central goal of the project, he and his coworkers have not yet established procedures for doing so. "That may involve a committee," he says, suggesting that sharing of this vast data set may prove to be a cumbersome process.
One potential roadblock comes about because copyright ownership is, in Lutz's words, "complicated." Unlike the arrangement that NASA made with IMAX, the raw footage of the seafloor does not remain the property of the government. Indeed, the various parties participating in the making of the film have conflicting copyright claims. Still, Lutz anticipates that proprietary interests will not in the end thwart the dissemination of information, something that would surely sour both investigators and NSF administrators. "I don't think anyone wants to go there," he says.
So for the moment, the name IMAX remains popular with both moviegoers and scientists. Indeed, investigators may soon be working closely with IMAX in another hostile realm: deep underground. A group of physicists led by Wick Haxton of the University of Washington in Seattle has recently mounted an effort to take over the Homestake Mine in western South Dakota and convert it into a giant laboratory for scientific research. Their $281 million proposal to NSF describes the various facilities the site would eventually house: particle detectors, an accelerator, workspaces, and, yes, the world's first underground IMAX theater.—David Schneider
American Scientist Comments and Discussion
To discuss our articles or comment on them, please share them and tag American Scientist on social media platforms. Here are links to our profiles on Twitter, Facebook, and LinkedIn.
If we re-share your post, we will moderate comments/discussion following our comments policy.